Table of Contents
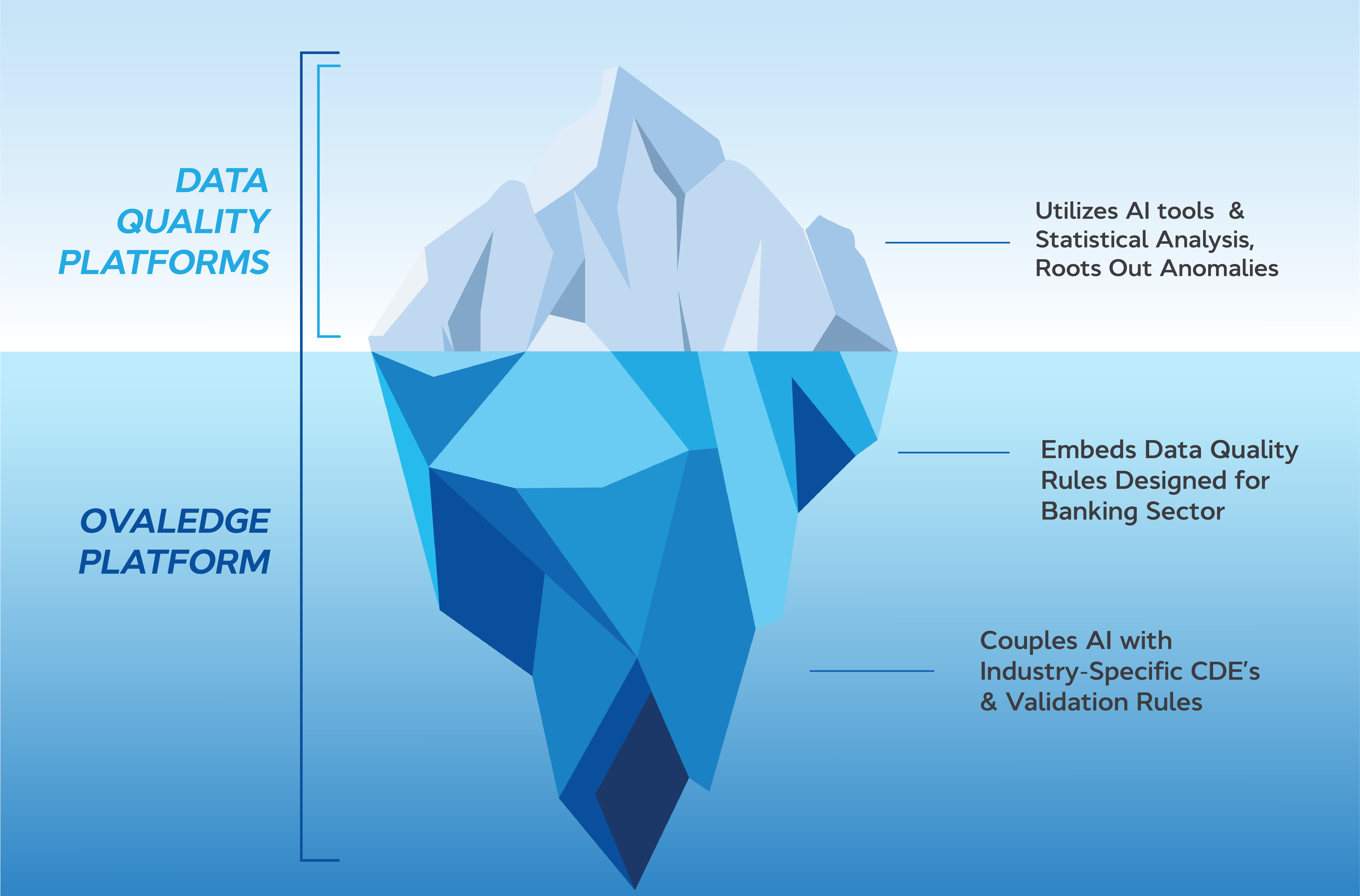
Data Quality Purpose-Built for Banking
For banks, issues with data quality can have dramatic repercussions financially and from a compliance perspective. In this blog, we’ll explore the reasons why high quality data is so important in finance, and how OvalEdge, with our industry-specific solution, can help you achieve it.
In 2012, one of the world’s biggest banks, HSBC Holdings (HSBC), was fined a staggering $1.9 billion plus $665 million in civil penalties by US regulators for failing to adhere to the strict guidelines around anti-money laundering (AML) laws. Inadvertently, the bank had been enabling illegal fund transfers and currency exchanges.
One of the core factors in this scandal was poor data quality. HSBC failed to put in checks and balances to identify suspicious data points, which in no small part enabled these nefarious transactions.
However, not just the large banks suffer when data quality lapses. Data quality issues are also a significant pain point for regional banks and credit unions.
Need for Data Quality in Banking
Failing to ensure data quality can have a ripple effect across a bank. Low data quality impacts privacy compliance, with users making more frequent mistakes. This is a significant failure for a business operating within one of the world’s most highly regulated industries and dealing with a vast volume of sensitive data.
However, privacy compliance is just the tip of the iceberg. Regional banks and credit unions must adhere to various reporting compliance regulations too, with laws covering fair lending, customer data safeguarding, enabling customers to make informed decisions, accurate disclosure, anti-money laundering, and capital adequacy. Failure to do so can lead to severe fines and reputational damage.
Beyond compliance, inadequate data quality can lead to poor decision-making. This is particularly important when it comes to managing risk. Banks need to have the highest quality data at their disposal to manage risk effectively, which is, of course, one of the most critical functions the organization must undertake.
Furthermore, improved data quality leads to better decisions in other areas too, including sales and marketing. When you have access to high-quality customer data, you can accurately target customers with products and services they are likely to want and that will ultimately improve their experience with your organization.
Finally, poor data quality hurts productivity. When data quality is low, general operations are less efficient. For example, verifying that the data you need is accurate takes longer. If not, errors could occur during billing, transaction processing, and customer experience processes.
Related Post: Data Governance and Data Quality: Working Together
Existing Data Quality Platforms are Not Purpose-Built for Banking
Several solutions are available to tackle the various data issues that arise when quality is poor. These solutions primarily use AI tools and statistical analysis to assess and fix data quality issues, rooting out anomalies.
However, these tools lack the specificity of a solution developed for the banking sector and, crucially, fail to address two other aspects of data quality: operational failure modes and compliance-specific failure modes.
An operational failure occurs when the stakeholders responsible for inputting or amending data make mistakes.
For example, a user may fail to log the correct address for a customer. They may include multiple addresses, multiple property numbers, miss street names, or unwittingly submit placeholder addresses.
Compliance-specific failures in a banking environment relate to data quality issues that impact regulatory guidelines.
For example, from a compliance standpoint, data quality issues can arise when reporting a customer’s income. Strict guidelines dictate how income should be captured and calculated, and if these guidelines aren’t followed, this is considered a compliance failure.
Existing tools that aren’t purpose-built for regional banks and credit unions, fail to address these critical data quality aspects. They use AI to map, clean, and send data to downstream applications. OvalEdge is different. Our tool covers every aspect of data quality by embedding data quality rules designed for the banking sector.
Related Posts:
1. AI Needs Domain Knowledge to Boost Data Quality
2. Data Quality Challenges for Fair Lending Compliance in Banking
OvalEdge: A Purpose-Built Data Quality Solution for Banks
OvalEdge has the AI and ML technologies that other solutions use but enriches these tools by embedding domain knowledge from the banking sector. Ultimately, OvalEdge is a data quality solution with pre-built domain knowledge embedded into the platform.
That's how we can move beyond hunting for data anomalies to identifying specific operational errors in context and catching compliance issues before you submit your reports to auditors. OvalEdge couples AI with industry-specific common data elements (CDEs) and validation rules based on banks' core operational and compliance requirements.
Critical data quality concerns are addressed because domain-specific validation rules are embedded into the product. You'll always be aware of the state of data quality in your institution, and alerts will be administered whenever an error is identified. This, paired with an analysis of the downstream impact of any errors, gives you a holistic view of data quality across every aspect.
Related Case Study: Improving Data Quality at a Regional Bank