Table of Contents
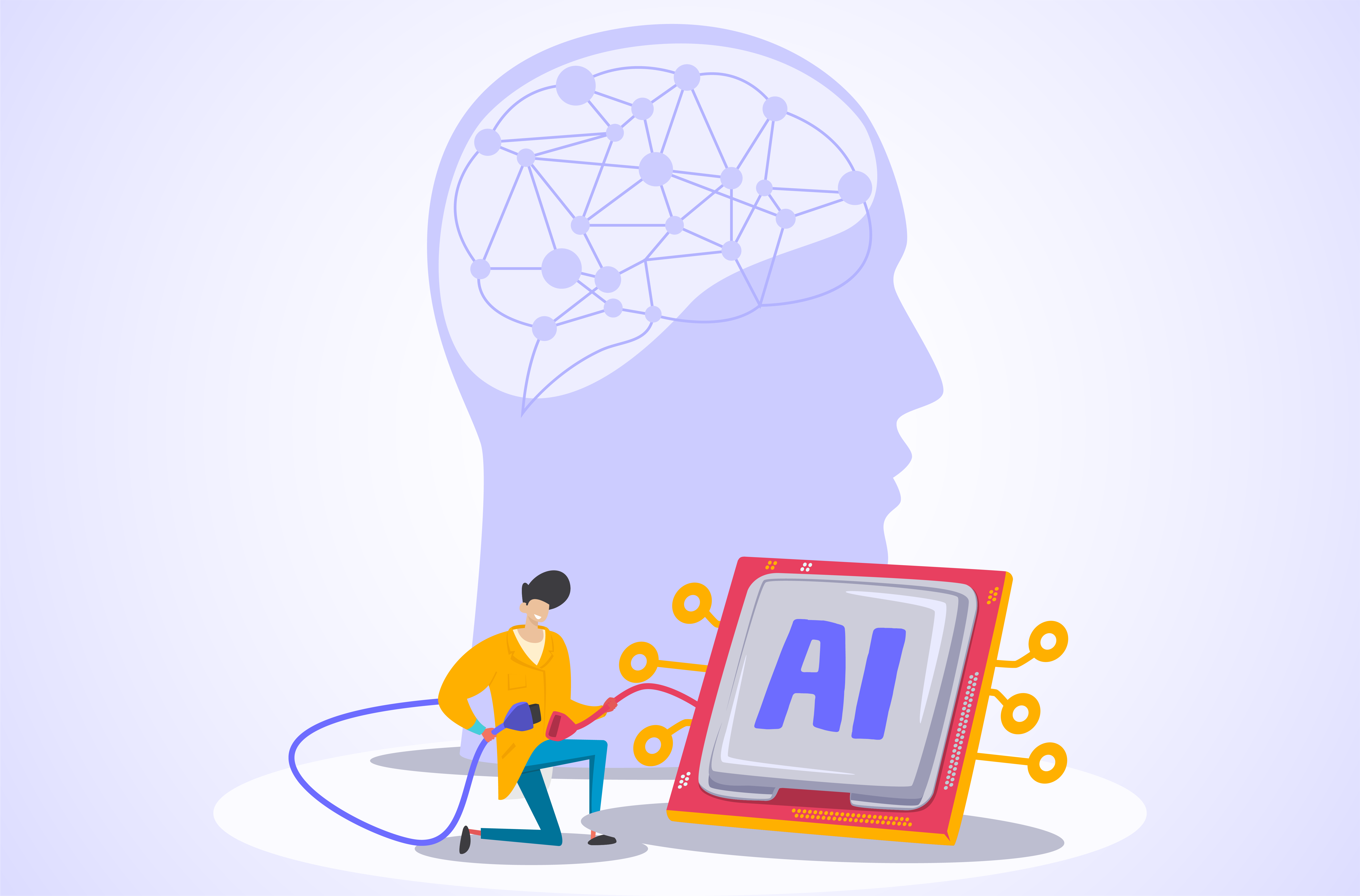
AI Needs Domain Knowledge to Boost Data Quality
In this article, we explain how AI and domain knowledge combined tackle every aspect of data quality and how OvalEdge provides this holistic solution for its banking customers.
AI alone cannot ensure robust data quality.
Without complementing AI with the domain knowledge specific to the banking industry, you’ll miss fixing the operational and compliance-specific errors that compromise data quality.
In this article, we’ll demonstrate how.
Strengths and Limitations of AI for Data Quality
In today’s IT environments, where banks produce and handle unprecedented volumes of data, AI is integral to ensuring data quality.
AI Excels in Fixing Nonsensical Data
AI tools are incredibly adept at detecting statistical data anomalies, such as data fields with missing or nonsensical values.
Take the FICO Score as an example. The FICO Score is measured between 300 and 850. If a score doesn't fall within this range, AI can be programmed to issue an alert. In another example, if an account holder’s Age shows as 190 years, this nonsensical value outlier will be flagged, and stakeholders will be alerted to take further action.
Related Post: 4 Steps to AI-Ready Data
Yet, AI Cannot Capture Operational Data Errors
However, AI often does not capture data quality issues arising from operational errors. That's because AI does not have the operational context to make a judgment to correct an issue if a mistake is made.
For example, if there is no standard rule for capturing a loan applicant's income on a weekly, monthly, or annual basis, the recorded income will vary. This is an operational error that AI cannot fix as the technology has no way of knowing the operational context in which customers report incomes in various ways. Data entries to loan systems are not often standardized.
In another example, bank staff often fail to record the correct loan amount after changes have been made during the application process. Instead of recording the changed loan amount, the base loan amount is captured even though the figure has altered after appraisals and counter offers. This data quality error can only be caught by manually embedding an alert that the changed loan should be captured in the systems. This requires operational knowledge to be embedded in the data systems.
AI Cannot Catch Compliance-Specific Data Errors Either
Beyond operational errors, there are instances where data quality errors arise because the data is not captured in compliance-specific formats. For example, GMI data must be captured in compliance-specific formats. Yet, many lenders struggle to ensure that staff adhere to this formatting when recording these data points. AI cannot fix such errors and require compliance-specific knowledge to be embedded in the data systems.
Furthermore, there are often errors in capturing the purpose of a loan, particularly when it comes to applications for refinancing non-mortgage-secured debt. Lenders must adhere to specific terminology that matches the HMDA’s definitions, but the terms used don't always concur. Such compliance-specific errors can be fixed using AI tools.
To Ensure Robust Data Quality, AI Needs To Be Complemented With Domain Knowledge
To ensure robust data quality that covers standard, operational, and compliance-specific errors, banks must look for tools with AI capabilities embedded in domain knowledge.
This approach ensures that you have a robust strategy to find and fix all of your data quality issues and a steadfast toolkit to boost the quality of your data over time.
Related Post: Data Quality Purpose-Built for Banking
Wrap Up
Our end-to-end data governance solution is the only platform that offers AI and ML technologies along with embedded domain knowledge specific to the banking sector.
With OvalEdge, AI works alongside human intelligence. We introduce industry-specific common data elements (CDEs) and validation rules based on the core practices of banking institutions that enable data quality improvement processes to complement the forensic search for data anomalies performed by our AI tools.
Because domain-specific validation rules are embedded into the product, you won't miss any operational or compliance-specific data quality issues. Using OvalEdge, you get the best of both worlds: a powerful AI-driven platform that continuously hunts for data quality issues and a purpose-built, industry-specific toolkit that uses human intelligence to uncover the errors AI can't.
Read Case Study: Improving Data Quality at a Regional Bank
OvalEdge recognized as a leader in data governance solutions
.png?width=1081&height=173&name=Forrester%201%20(1).png)
“Reference customers have repeatedly mentioned the great customer service they receive along with the support for their custom requirements, facilitating time to value. OvalEdge fits well with organizations prioritizing business user empowerment within their data governance strategy.”
.png?width=1081&height=241&name=KC%20-%20Logo%201%20(1).png)
“Reference customers have repeatedly mentioned the great customer service they receive along with the support for their custom requirements, facilitating time to value. OvalEdge fits well with organizations prioritizing business user empowerment within their data governance strategy.”
Gartner, Magic Quadrant for Data and Analytics Governance Platforms, January 2025
Gartner does not endorse any vendor, product or service depicted in its research publications, and does not advise technology users to select only those vendors with the highest ratings or other designation. Gartner research publications consist of the opinions of Gartner’s research organization and should not be construed as statements of fact. Gartner disclaims all warranties, expressed or implied, with respect to this research, including any warranties of merchantability or fitness for a particular purpose.
GARTNER and MAGIC QUADRANT are registered trademarks of Gartner, Inc. and/or its affiliates in the U.S. and internationally and are used herein with permission. All rights reserved.
.png?width=1081&height=173&name=Forrester%201%20(1).png)
“Reference customers have repeatedly mentioned the great customer service they receive along with the support for their custom requirements, facilitating time to value. OvalEdge fits well with organizations prioritizing business user empowerment within their data governance strategy.”
.png?width=1081&height=241&name=KC%20-%20Logo%201%20(1).png)
“Reference customers have repeatedly mentioned the great customer service they receive along with the support for their custom requirements, facilitating time to value. OvalEdge fits well with organizations prioritizing business user empowerment within their data governance strategy.”
Gartner, Magic Quadrant for Data and Analytics Governance Platforms, January 2025
Gartner does not endorse any vendor, product or service depicted in its research publications, and does not advise technology users to select only those vendors with the highest ratings or other designation. Gartner research publications consist of the opinions of Gartner’s research organization and should not be construed as statements of fact. Gartner disclaims all warranties, expressed or implied, with respect to this research, including any warranties of merchantability or fitness for a particular purpose.
GARTNER and MAGIC QUADRANT are registered trademarks of Gartner, Inc. and/or its affiliates in the U.S. and internationally and are used herein with permission. All rights reserved.
.png?width=1081&height=173&name=Forrester%201%20(1).png)
“Reference customers have repeatedly mentioned the great customer service they receive along with the support for their custom requirements, facilitating time to value. OvalEdge fits well with organizations prioritizing business user empowerment within their data governance strategy.”
.png?width=1081&height=241&name=KC%20-%20Logo%201%20(1).png)
“Reference customers have repeatedly mentioned the great customer service they receive along with the support for their custom requirements, facilitating time to value. OvalEdge fits well with organizations prioritizing business user empowerment within their data governance strategy.”
Gartner, Magic Quadrant for Data and Analytics Governance Platforms, January 2025
Gartner does not endorse any vendor, product or service depicted in its research publications, and does not advise technology users to select only those vendors with the highest ratings or other designation. Gartner research publications consist of the opinions of Gartner’s research organization and should not be construed as statements of fact. Gartner disclaims all warranties, expressed or implied, with respect to this research, including any warranties of merchantability or fitness for a particular purpose.
GARTNER and MAGIC QUADRANT are registered trademarks of Gartner, Inc. and/or its affiliates in the U.S. and internationally and are used herein with permission. All rights reserved.