Table of Contents
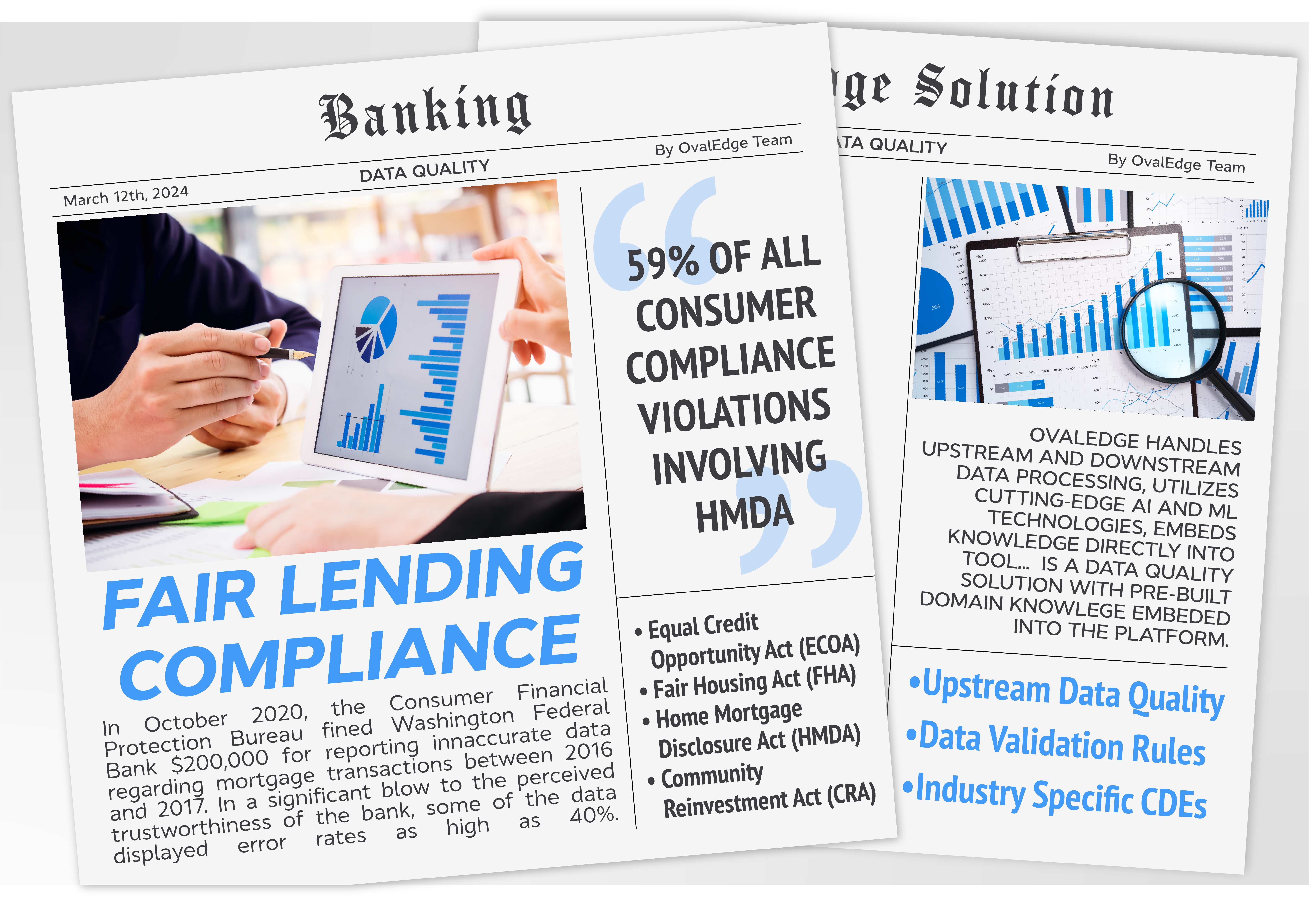
Implementing Data Quality for Fair Lending Compliance in Banking
Did you know that fair lending compliance is one of the biggest pain points for regional banks and credit unions? In recent years, regulatory agencies have vigorously ramped up fair lending investigations. This is because around 45 million Americans face barriers to accessing affordable credit, and regional banks and credit unions feel the repercussions of non-compliance.
During our research, one participant, a Compliance and Fair Lending Officer with 19 years of experience in the sector, told us that regional banks and credit unions are 30% more likely to face fair lending compliance issuess.
To date, the banking sector has become incredibly apt at using data-driven initiatives to ensure the smooth and efficient running of everyday processes. They've had to. Even calculating a dollar short account balance is a fundamental error with drastic consequences.
Yet, while data-driven accounting processes are incredibly robust, the same can't always be said for auxiliary processes, like ensuring non-discriminatory lending. Although often overlooked in place of privacy compliance, fair lending compliance regulations are a vital concern for regional banks, credit union, and community banks.
Related Post: Risk Assessment in Banking
Key Fair Lending Regulations
Banks in the US must comply with a series of regulations to ensure fair lending practices. They include the following:
- Equal Credit Opportunity Act (ECOA)
- Fair Housing Act (FHA)
- Home Mortgage Disclosure Act (HMDA)
- Community Reinvestment Act (CRA)
Fair lending compliance violations are those most cited by the US Federal Reserve, with 59 percent of all consumer compliance violations involving HMDA. In fact, the community bank and credit union representatives that we interviewed identified data reporting requirements related to loan applicants and loan terms under the HMDA as one of three regulatory areas that most burden their organization.
Violation of fair lending compliance regulations leads to fines and, more importantly, reputational damage. Even if penalties are manageable, the impact on a bank's reputation often isn't. For example, in October 2020, the Consumer Financial Protection Bureau fined Washington Federal Bank $200,000 for reporting inaccurate data regarding mortgage transactions between 2016 and 2017. This violated the HMDA and the Consumer Financial Protection Act of 2010 (CFPA). In a significant blow to the perceived trustworthiness of the bank, some of the data displayed error rates as high as 40%.
That's why communicating and ensuring data quality in fair lending compliance reporting is essential. When it's not present, it leads to multiple errors, such as the wrong income reported for loan applications, incorrect addresses or multiple addresses listed, loan amounts inaccurately captured, and skewed rate lock dates.
The fundamental driver in ensuring banks file their reports to auditors correctly is data quality.
Common Data Quality Issues
Several data quality issues commonly affect regional banks when it comes to ensuring fair lending compliance. They include operational errors, such as:
- Failure to capture the correct income for potential borrowers with multiple revenue streams
- Neglecting to change rate-lock dates when rates are re-locked
- Inputting incorrect property location data
- Base loan amounts captured and not updated loan amounts in the event of a change of circumstance
And there are issues with data formats and definitions that have a direct impact on compliance, such as:
- Failure to comply with policies and definitions for GMI data, such as ethnicity, race, and sex
- Using terms for actions such as refinancing that don't match regulated definitions
Data Quality Tool for Fair Lending Compliance
Most data quality vendors aren't purpose-built for banking compliance, so they aren't good enough to support the complexities facing regional banks. Instead, these solutions focus on automatically mapping data, cleaning it, and providing it to downstream applications using AI and ML techniques.
Conversely, platforms purpose-built for compliance that embed detailed domain knowledge are point providers, they work reactively downstream and generally lack the proactive capabilities required to identify data anomalies upstream.
OvalEdge handles upstream and downstream data processing, utilizes cutting-edge AI and ML technologies, embeds domain knowledge directly into the tool, and includes a range of dedicated features designed for fair lending compliance. OvalEdge is a data quality solution with pre-built domain knowledge embedded into the platform.
AI alone is unlikely to catch compliance infringements specific to fair lending. OvalEdge pairs AI with industry-specific common data elements (CDE) and validation rules. The platform's data validation rules are based on banks' core operational and fair lending compliance requirements when reporting to auditors.
Related Post: 4 Steps to AI-Ready Data
OvalEdge's dedicated validation rules address key pain points across various areas, such as income, when gross income is captured incorrectly, miscalculated rate spread, and GMI data, where lenders fail to comply with the correct policies for recording these data points.
With OvalEdge, lenders can rest assured that these critical data quality concerns are addressed because domain-specific validation rules are embedded into the product.
Users are alerted whenever an infringement is identified and given an analysis of the downstream impact. Ultimately, OvalEdge is purpose-built for fair lending compliance.
Book a call with us to find out1. How OvalEdge can help you achieve fair lending compliance for every audit- quickly, simply, and cost-effectively. 2. The most efficient way to develop and implement a framework for data quality improvement. 3. How OvalEdge supports a progressive approach to data governance, adapting to your needs and use cases as and when they emerge. |
Schedule a Demo
Fill the information below to set up a demo.