Table of Contents
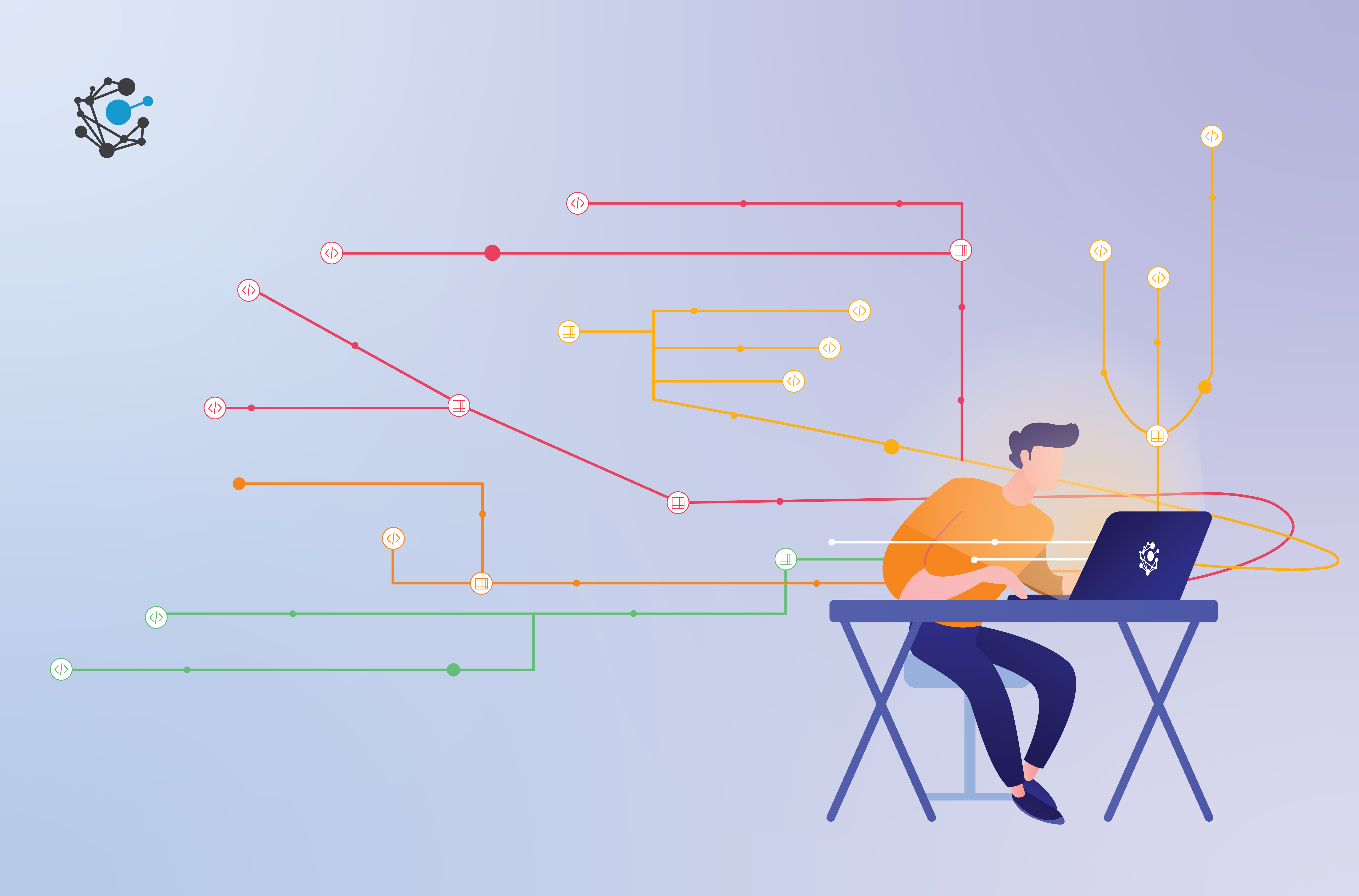
Data Lineage | Drivers and Techniques
Data lineage, tracking, and recording the various processes and changes data has been through since its inception is one of the core aspects of data governance. It enables organizations to fully understand how data flows from one place to another within a cloud-based or on-prem infrastructure, tracking its course through databases, data lakes, ETLs, data warehouses, and reporting systems.
However, data lineage is more than just an additional element to a data governance tool kit; it is a regulatory requirement. In this blog, we'll examine the core outcomes of a comprehensive data lineage program before explaining the technical processes involved in building data lineage.
Drivers of Data Lineage
Why is data lineage so high on the data governance agenda? Today, companies collect and utilize data at a staggering rate in the era of big data. Gone are the days when BI involved targeted data sets; instead, data analysis has become industrialized.
And for a good reason. Advances in AI and other technologies enable data-driven insights to inform and influence every aspect of a business, providing companies with countless opportunities to gain a competitive edge.
However, for this process to work, users must trust the data available to them, and for that, they need to know where it came from, where it's been, and where it's going. And when you can't trace the lineage of your data, you can't determine its quality.
At the same time, maintaining data privacy compliance has become a top priority for companies in every sector. However, understanding where PII information has been and who has accessed it is necessary for the task.
The modern data ecosystem is a minefield. It’s a complex web of systems and processes that users can only navigate successfully with a dedicated governance tool.
Lack of trust in data products: Data-driven organizations only succeed when everyone is onboard and working towards a common goal. Yet, users are becoming increasingly disillusioned with data products because, without accurate lineage, there is no proof that they are what they claim to be.
Never-ending data quality issues: When you can't trace the origin and flow of data, you can't improve its quality. As a result, an absence of lineage leads to ongoing data quality issues.
Regulatory compliance: Data privacy compliance is just one of the many regulatory compliance statutes that impact businesses in every sector. Auditors need proof of data lineage to ensure it's been handled correctly.
Related Post: Benefits of Data Lineage
Data Lineage Techniques
There are three core ways of tracking data lineage: at the system, object, and column levels.
System level: Tracking data lineage at the system level enables data teams to see how data moves through various systems, from ERP systems to data warehouses to reporting systems.
The benefit of tracking data lineage at this level is that data architecture teams can quickly understand the overall state of data lineage in the organization. It's like a high-level overview.
Tracking Data Lineage at the System Level
Object level: In OvalEdge, tables, and report files are considered objects. The OvalEdge GUI depicts the lineage at the object level, which helps users communicate with the right people if there is a problem with the quality of data downstream.
You can quickly find everyone affected by a problem through impact analysis and inform and educate them about it. Object-level data lineage tracking is essential to building trust in data.
Tracking Data Lineage at the Object Level
Column level: All table columns, file columns, and report attributes are connected and displayed in OvalEdge. Column-level tracking is vital for compliance and impact analysis because it enables users to drill down to precise data points.
Tracking Data Lineage at the Column Level
Related Post: 3 Data Privacy Compliance Challenges that can be solved with Data Governance
How to Build and Visualize Data Lineage
There are two approaches to lineage building and visualization: the manual approach and the automated approach. While manual lineage building is incredibly complex, there are some key reasons why some organizations choose to do it.
Predominantly, organizations commit to manual lineage building to adhere to specific compliance requirements. However, automated lineage building is both simpler and more comprehensive.
A lineage tool automates lineage building by parsing the source code of various supported systems such as reporting systems, ETLs, data warehouses, and SQLs. After reverse engineering, lineage is built automatically. You can access data lineage via backend algorithms to automate various processes.
Many specific applications include lineage tracking facilities, but when users want to move data out of the application, they encounter difficulties. This is where a tool’s automated lineage-building facilities are critical.
Creating custom infrastructure is often required in today’s complex and data-driven business landscape. Consequently, data is moved regularly from place to place.
Conclusion
When it comes to data governance, certain aspects can’t be ignored. Data lineage is one of those aspects. Data lineage is essential to the governance process regardless of your industry or sector.
With so much data to govern, lineage tracking and building can be incredibly difficult and time-consuming.
What you should do now
|
Schedule a Demo
Fill the information below to set up a demo.